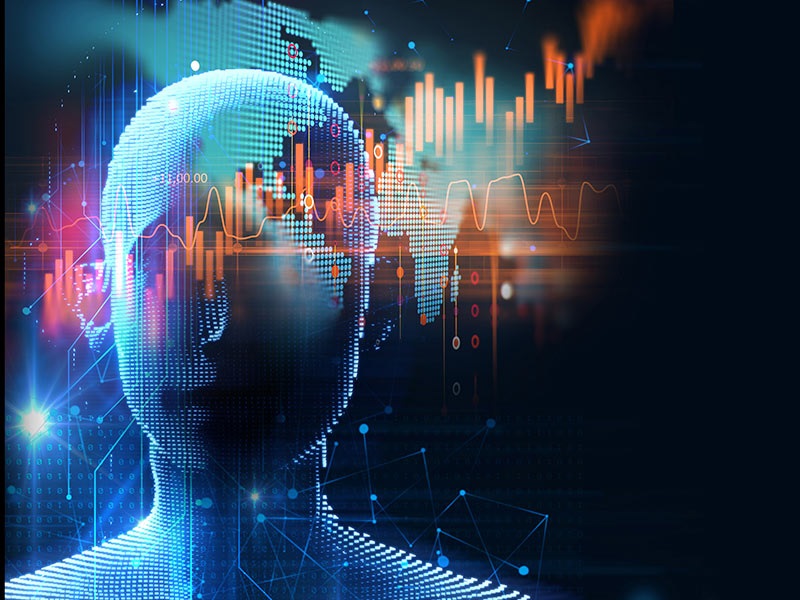
Since the beginnings of man-made reasoning, analysts have since a long time ago looked to test the knowledge of machine frameworks by having them mess around against people. It is generally expected idea that one of the signs of human insight is the capacity to think inventively, think about different potential outcomes, and remember a drawn out objective while settling on momentary choices. On the off chance that PCs can play troublesome games similarly just as people then doubtlessly they can deal with considerably more convoluted errands. From early checkers-playing bots created during the 1950s to the present profound learning-fueled bots that can beat even the best players on the planet at games like chess, Go and DOTA, machines that can observe answers for puzzles is just about as old as AI itself, if not more established.
Accordingly, it’s a good idea that one of the center examples of AI that associations create is the objective driven frameworks design. Like different examples of AI, we see this type of computerized reasoning used to take care of a typical arrangement of issues that would some way or another require human intellectual ability. In this specific example, the test that machines address is the need to track down the ideal answer for an issue The issue may be tracking down a way through a labyrinth advancing a production network, or advance driving courses and inactive time. Despite the particular need, the power that we’re searching for here is learning through experimentation, and deciding the most ideal way to tackle something, regardless of whether it’s not the most self-evident.
Support learning and learning through experimentation
One of the most charming, yet least utilized, types of AI is support learning. Rather than managed learning approaches in which machines learn by being prepared by people with all around marked information, or unaided learning approaches in which machines attempt to learn through revelation of bunches of data and different groupings, support learning endeavors to learn through experimentation, utilizing ecological input and general objectives to emphasize towards progress.
Without the utilization of AI, associations rely upon people to make projects and decides based frameworks that guide programming and equipment frameworks on the best way to work. Where projects and rules can be fairly powerful in overseeing cash, workers, time and different assets, they experience the ill effects of weakness and unbending nature. The frameworks are just pretty much as solid as the principles that a human makes, and the machine isn’t actually learning by any means. Rather, it’s the human knowledge joined into decides that makes the framework work.
Objective learning AI frameworks then again are given not very many principles, and have to figure out how the framework chips away at their own through emphasis. Along these lines, AI can completely streamline the whole framework and not rely upon human-set, fragile guidelines. Objective driven frameworks have demonstrated their value to show the uncanny capacity for frameworks to see as the “covered up rules” that tackle testing issues. It isn’t shocking exactly how valuable objective driven frameworks are in regions where asset improvement is an unquestionable requirement.
Computer based intelligence can be productively utilized in situation reenactment and asset enhancement. By applying this summed up way to deal with learning, AI-empowered frameworks can be defined to improve a specific objective or situation and track down numerous answers for arriving, some not even clear to their more-innovative human partners. Along these lines, while the objective driven frameworks design hasn’t considered a lot of execution as different examples like the acknowledgment, prescient examination, or conversational examples, the potential is similarly as tremendous across a wide scope of enterprises.